The recent FAT* conference in Barcelona was primarily designed to bring “together researchers and practitioners interested in fairness, accountability, and transparency in socio-technical systems“. As the conference is run by the ACM I went in expecting the vast majority of speakers and attendees to be from a computer science background. To the communities benefit, the audience was very diverse in discipline. Ethics, politics and law researchers, disability and minority advocacy groups, and non-government development agencies were all very well represented both delivering talks and in the corridor conversations.
I came away with some key learning’s, listed here in no particular order-
- The algorithmic definitions of fairness and the conceptual definitions of fairness need a common language. At the moment there is a lot of groups talking past each other with mismatched terminology.
- Fairness, as a concept, is usually framed in computer science as a machine learning issue. This does not match with the understanding of the wider audience, who view all decision making as in scope. Decision trees, fuzzy logic, recommendation engines, all of it.
- Everyone is hooked on Fairness. It is getting more attention than transparency or accountability
- There was almost no Computer science based accountability conversations, all were legal and ethical. This is (in my opinion) a good thing, it’s beyond the scope of what would be considered a CS problem. However, this could result in a rude awaking for engineers when we start seeing laws related to who is responsible.
- Transparency was discussed frequently. Disappointingly, most of the conversations are focused on model debugging and the ability to identify important variables, primarily for an engineering and researcher audience. This is important for the functional aspect of getting applications using ML running but does not solve the issues of interpretability. I anticipate be a wave of work in the HCI literature to understand the best approach to explain and visualise Machine Learning models for non technical audiences.
- A common theme throughout the conference was the significant criticism pointed towards the industry and government entities. A lot of this targeted “Ethics washing”, a term describing the ethics frameworks companies create and apply to themselves with no external oversight or accountability. These are seen as a veil companies give themselves to allow continued unethical work. A topic addressed in the recent paper – “Algorithmic Fairness from a Non-ideal Perspective” [1].
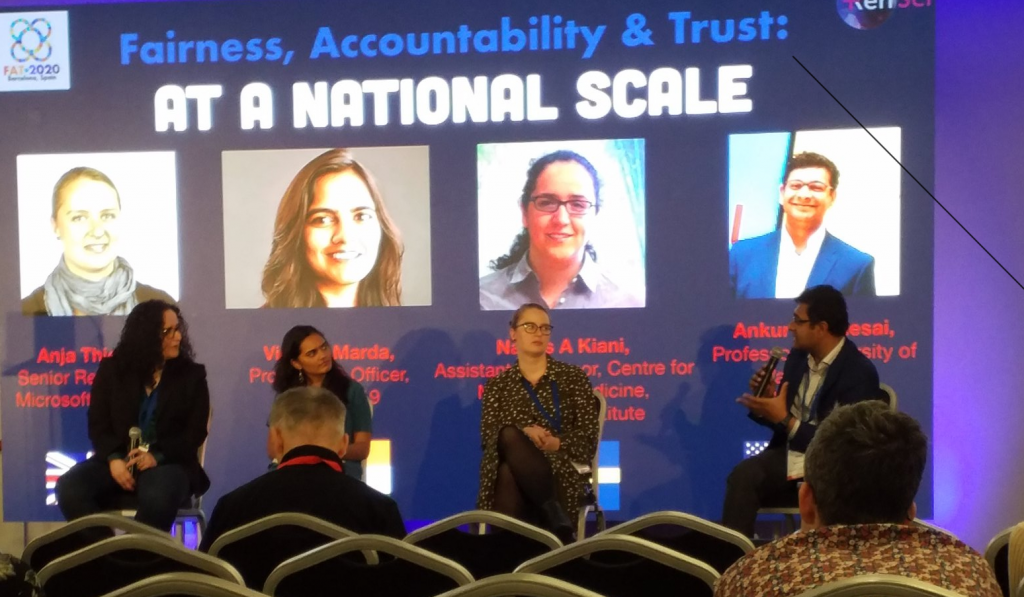
There was also some wider conversation on new, targeted, laws to ensure fair and safe decision making. Tho, as Vidushi Marda (On twitter: @VidushiMarda) casually mentioned, new laws may not be required, we need to enforce existing laws including the UN charter of human rights.
Mental health was almost completely missing from the conversations, only mentioned during one of the workshops. Only two talks (of 95+) addressed healthcare [2],[3]. One that reviews the explainability of clinical data and the other detailing the roll-out of a sepsis detection tool in a real-world environment. Both worth a read.
Four things that I am encouraging people to read that came up from the conference-
- Doctor XAI: an ontology-based approach to black-box sequential data classification explanations-https://dl.acm.org/doi/pdf/10.1145/3351095.3372855?download=true
- FlipTest: Fairness Testing via Optimal Transport-https://dl.acm.org/doi/pdf/10.1145/3351095.3372845?download=true
- And Ayanna Howard’s keynote, you can find her work on excessive trust here –https://rh.gatech.edu/news/507361/emergencies-should-you-trust-robot
- GDPR Articles 22 & 9
——————-
[1] Fazelpour, S., & Lipton, Z. C. (2020). Algorithmic Fairness from a Non-ideal Perspective. (i), 1–14.
[2] Sendak, M., Elish, M., Gao, M., Futoma, J., Ratliff, W., Nichols, M., … O’Brien, C. (2019). “The Human Body is a Black Box”: Supporting Clinical Decision-Making with Deep Learning. 99–109. Retrieved from http://arxiv.org/abs/1911.08089
[3] Panigutti, C., Perotti, A., Pedreschi, D., & Pedreschi, D. 2020. (2020). Doctor XAI: An ontology-based approach to black-box sequential data classification explanations. 629–639. https://doi.org/10.1145/3351095.3372855